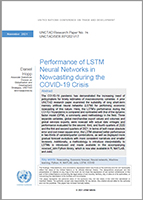
The COVID-19 pandemic has demonstrated the increasing need of policymakers for timely estimates of macroeconomic variables.
A prior UNCTAD research paper examined the suitability of long short-term memory artificial neural networks (LSTM) for performing economic nowcasting of this nature. Here, the LSTM's performance during the COVID-19 pandemic is compared and contrasted with that of the dynamic factor model (DFM), a commonly used methodology in the field.
Three separate variables, global merchandise export values and volumes and global services exports, were nowcast with actual data vintages and performance evaluated for the second, third, and fourth quarters of 2020 and the first and second quarters of 2021.
In terms of both mean absolute error and root mean square error, the LSTM obtained better performance in two-thirds of variable/quarter combinations, as well as displayed more gradual forecast evolutions with more consistent narratives and smaller revisions.
Additionally, a methodology to introduce interpretability to LSTMs is introduced and made available in the accompanying nowcast_lstm Python library, which is now also available in R, MATLAB, and Julia.